DataOps solutions have become essential for automating, orchestrating, and scaling data pipelines. With numerous tools available, it’s crucial to select one that aligns with your organization’s unique needs. Here’s a comparison of some of the most popular DataOps solutions, covering their features, pros, and cons.
1. Apache Airflow
- Overview: Apache Airflow is a leading open-source DataOps tool known for its workflow automation capabilities, especially for complex data pipelines.
- Features:
- Workflow management via Directed Acyclic Graphs (DAGs)
- Integration with various data sources
- Extensibility with custom operators
- Pros:
- Highly flexible and adaptable
- Strong community support
- Excellent for Python-centric workflows
- Cons:
- Limited real-time capabilities
- Higher setup complexity for beginners
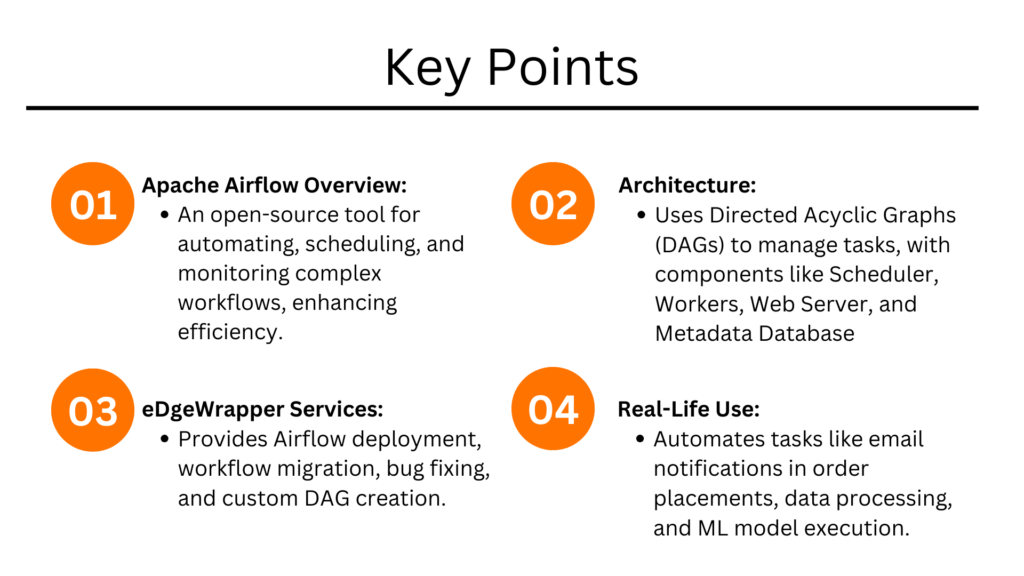
2. dbt (Data Build Tool)
- Overview: dbt is a transformation-focused DataOps tool, making it ideal for managing ETL and ELT pipelines directly within a data warehouse.
- Features:
- SQL-based data transformations
- Data testing and lineage tracking
- Version control for data models
- Pros:
- Excellent for SQL transformations
- Strong focus on data lineage and testing
- Integrates well with cloud data warehouses
- Cons:
- Limited functionality for data ingestion and orchestration
- Requires SQL proficiency for effective use
3. Talend
- Overview: Talend is an end-to-end DataOps platform with features for data integration, governance, and quality, tailored for enterprise use.
- Features:
- Comprehensive data integration tools
- Real-time data quality and governance
- Support for multi-cloud and on-premises environments
- Pros:
- Excellent data quality and governance features
- Comprehensive toolset for data management
- Robust integration options
- Cons:
- High licensing costs for enterprise solutions
- Requires additional training for complex configurations
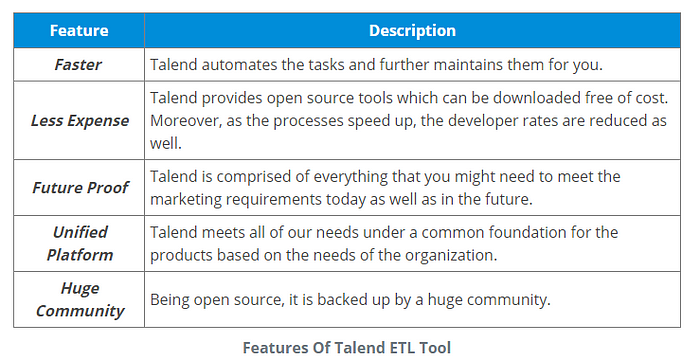
4. Prefect
- Overview: Prefect provides a Python-based DataOps platform that allows for building, orchestrating, and monitoring data workflows with resilience.
- Features:
- Built-in error handling and task retries
- API-driven and cloud-native support
- Easy integration with Python-based workflows
- Pros:
- Simplifies complex workflows with a Pythonic approach
- Built-in fault tolerance and resilience
- Lightweight setup with cloud orchestration option
- Cons:
- Limited support for non-Python environments
- Smaller community compared to some established tools
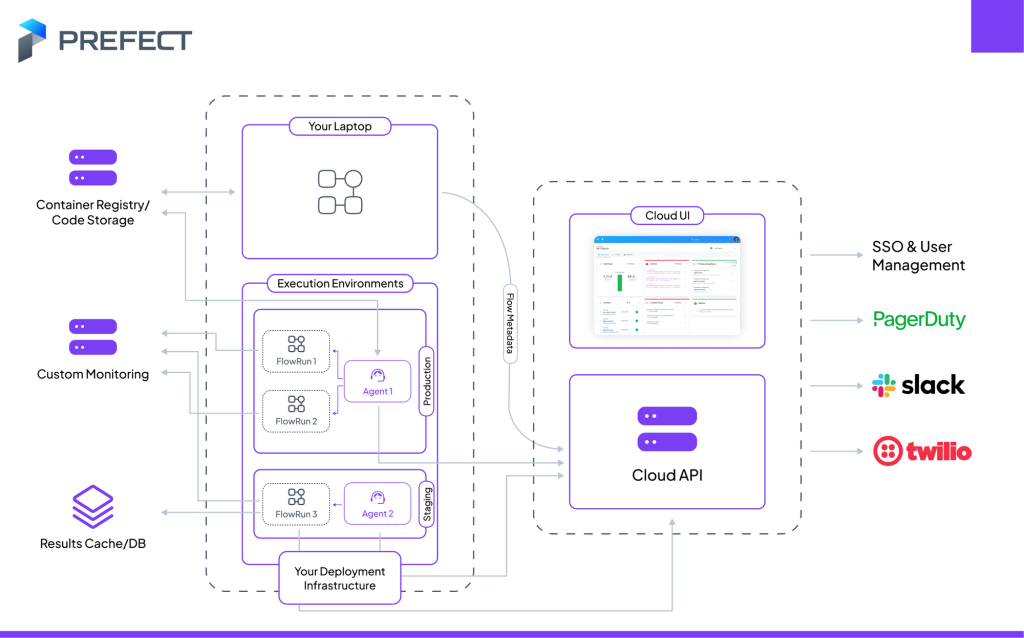
5. Fivetran
- Overview: Fivetran specializes in automating the data ingestion process by continuously syncing data from various sources to a data warehouse.
- Features:
- Fully managed connectors for popular data sources
- Automated schema mapping
- Real-time data integration
- Pros:
- Minimal setup and maintenance required
- Automated schema updates
- Reliable data synchronization
- Cons:
- Limited transformation capabilities
- High costs for larger data volumes