DataOps is a set of practices that combine software engineering principles with data management to increase the speed and quality of data delivery. By automating data pipelines, improving collaboration, and reducing time to market, DataOps empowers organizations to make data-driven decisions faster.
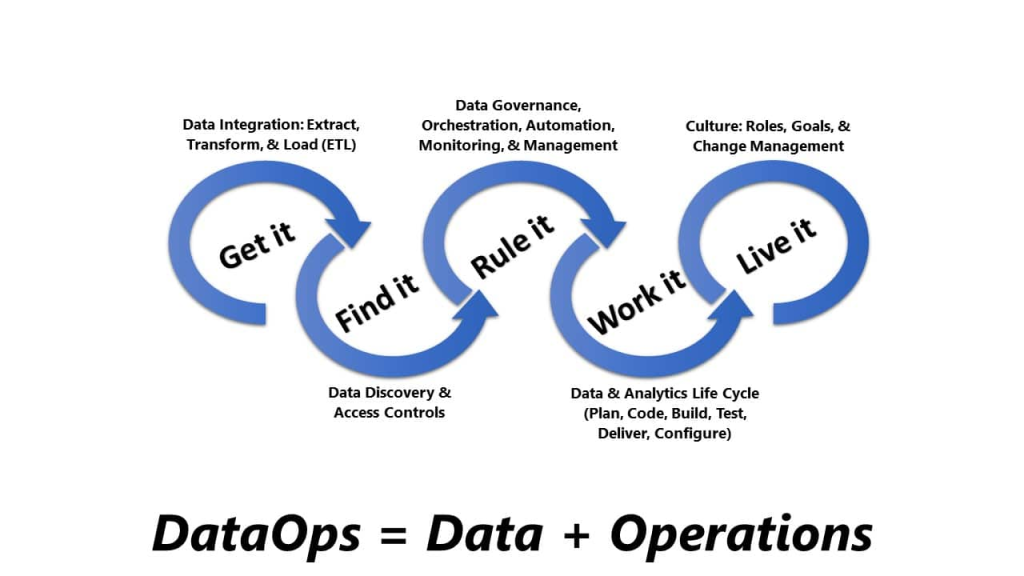
Core Principles of DataOps
- Continuous Integration and Continuous Delivery (CI/CD): Automate data pipelines to ensure consistent and reliable data flows.
- Collaboration and Communication: Foster collaboration between data engineers, data scientists, and business analysts.
- Data Quality and Monitoring: Implement data quality checks and monitoring to ensure data accuracy and reliability.
- Self-Service Data: Empower business users to access and analyze data independently.
Implementing DataOps for Continuous Integration and Delivery
1. Define Your DataOps Goals:
- Identify Pain Points: Determine the specific challenges your organization faces in data delivery.
- Set Clear Objectives: Define what you want to achieve with DataOps, such as faster time to insights, improved data quality, or increased data accessibility.
- Establish a DataOps Team: Assemble a cross-functional team of data engineers, data scientists, and business analysts.
2. Choose the Right Tools and Technologies:
- Data Orchestration: Use tools like Apache Airflow, Luigi, or dbt to automate data pipelines.
- Data Integration: Employ tools like Talend, Informatica, or Fivetran for data ingestion and transformation.
- Data Warehousing and Data Lakes: Utilize data warehouses or data lakes like Snowflake, Databricks, or Amazon Redshift.
- Data Quality: Implement data quality tools like Great Expectations or Data Quality.
- CI/CD: Implement CI/CD pipelines with tools like Jenkins, GitLab CI/CD, or CircleCI.
3. Establish Data Governance and Standards:
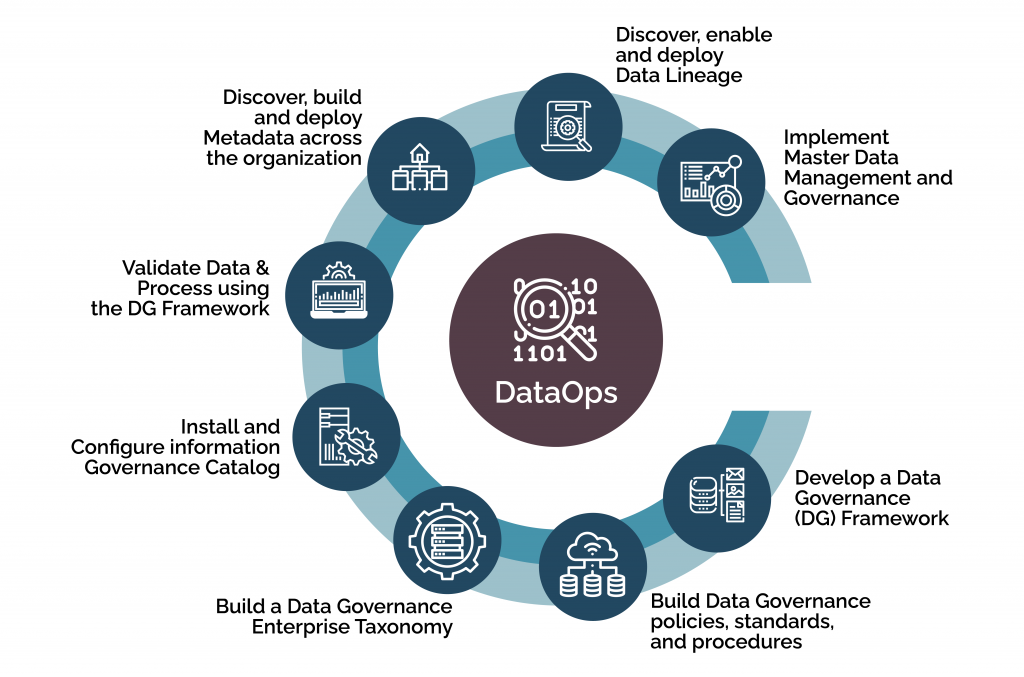
- Data Governance: Define data ownership, data quality standards, and data security policies.
- Data Catalog: Create a data catalog to document data assets and their metadata.
- Data Lineage: Track the origin and transformation of data to improve data understanding and troubleshooting.
4. Implement Data Pipelines:
- Design Data Pipelines: Break down complex data pipelines into smaller, modular components.
- Automate Data Ingestion: Set up automated data ingestion processes from various sources.
- Transform and Clean Data: Use data transformation tools to clean, enrich, and transform data.
- Load Data into the Target System: Load transformed data into data warehouses, data marts, or data lakes.
5. Monitor and Optimize:
- Implement Monitoring: Use tools like Prometheus and Grafana to monitor data pipeline performance.
- Set Up Alerts: Define alerts for failures, anomalies, and performance degradation.
- Optimize Data Pipelines: Continuously optimize data pipelines for performance and efficiency.
6. Foster a Data-Driven Culture:
- Data Literacy: Educate employees on data concepts and tools.
- Self-Service Analytics: Empower business users to access and analyze data independently.
- Data-Driven Decision Making: Encourage data-driven decision-making at all levels of the organization.
Key Benefits of DataOps
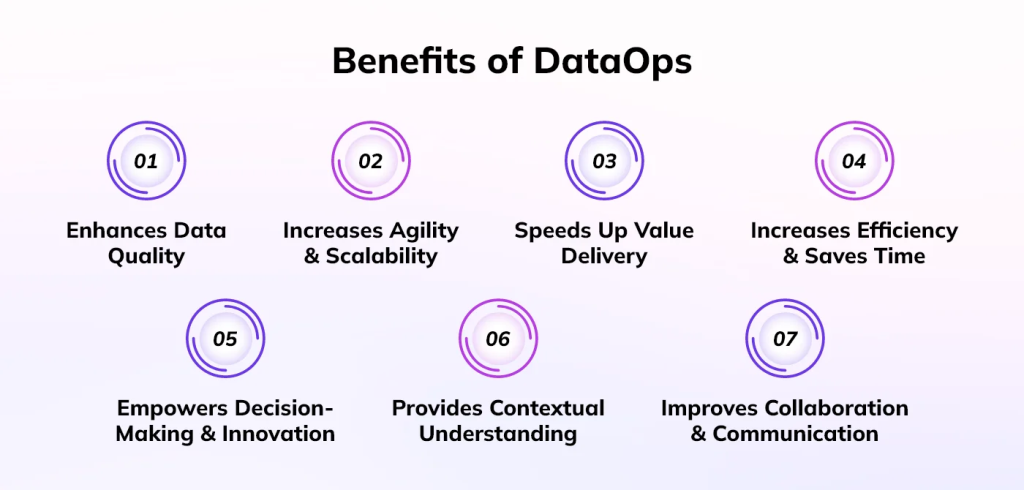
By implementing DataOps, organizations can achieve the following benefits:
- Increased Data Velocity: Faster data delivery and time to insights.
- Improved Data Quality: Higher data accuracy and reliability.
- Enhanced Collaboration: Better collaboration between data teams.
- Reduced Time to Market: Faster deployment of data products.
- Increased Innovation: Empowers data scientists and analysts to focus on innovation.