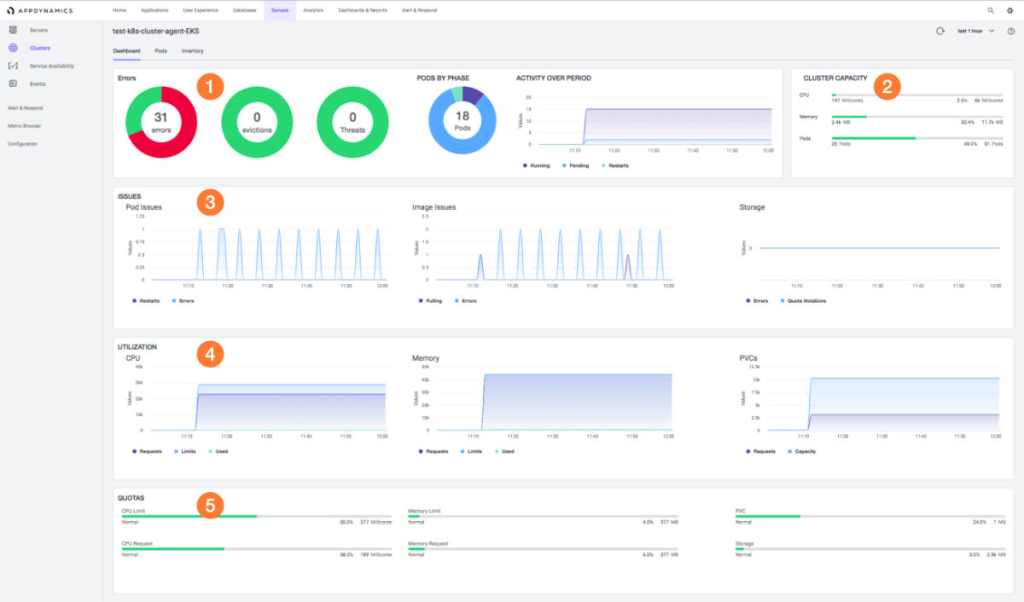
How AppDynamics is Revolutionizing Monitoring and Observability with MLOps
Are you tired of traditional monitoring and observability tools that only provide limited insights into your application performance? Look no further than AppDynamics, the leading application performance monitoring solution that uses MLOps to provide real-time, actionable insights into your application’s health.
What is MLOps?
Before we dive into how AppDynamics is using MLOps, let’s first define what it is. MLOps, or Machine Learning Operations, is the practice of incorporating machine learning into the software development lifecycle. It involves the integration of automated machine learning pipelines, continuous integration and deployment, and monitoring and observability tools to streamline the development and deployment of machine learning models.
AppDynamics’ Approach to MLOps
AppDynamics has taken a unique approach to MLOps, leveraging its powerful monitoring and observability platform to provide real-time insights into the performance of machine learning models. By integrating machine learning models into its platform, AppDynamics is able to provide a holistic view of your application, including the performance of your machine learning models.
One of the key benefits of AppDynamics’ approach to MLOps is its ability to identify anomalies and issues with your machine learning models in real-time. By leveraging its powerful machine learning algorithms, AppDynamics is able to detect issues with your models before they impact your application’s performance.
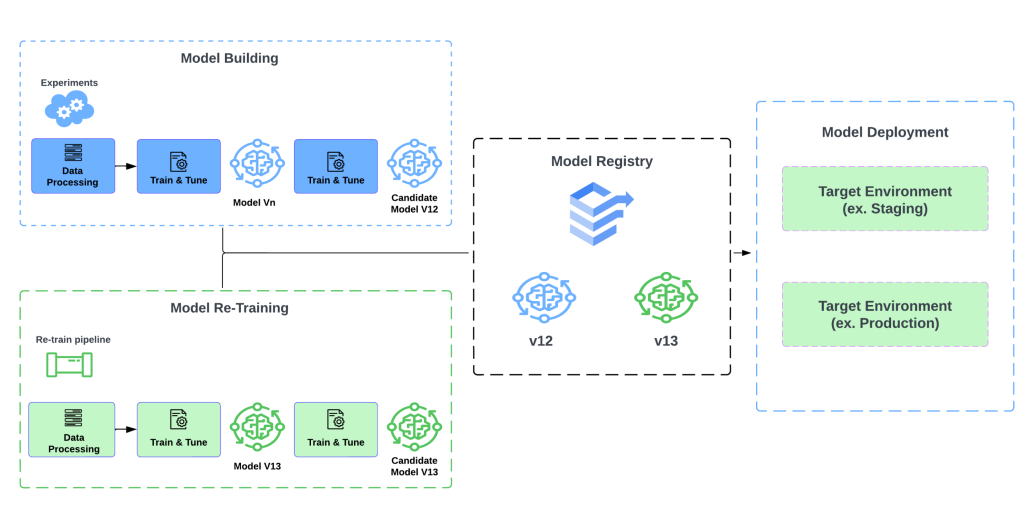
Real-World Examples
To better understand how AppDynamics is using MLOps in monitoring and observability, let’s take a look at some real-world examples.
Example 1: Fraud Detection
One of the most common use cases for machine learning is fraud detection. However, traditional fraud detection tools can be limited in their ability to detect new and emerging fraud patterns. By leveraging AppDynamics’ MLOps platform, companies can continuously monitor their fraud detection models and identify new patterns and trends in real-time.
Example 2: Predictive Maintenance
Predictive maintenance is another area where AppDynamics’ MLOps platform can provide significant benefits. By integrating machine learning models into their monitoring and observability platform, companies can continuously monitor the health of their equipment and predict when maintenance is required. This can help companies reduce downtime and improve overall productivity.
Conclusion
In conclusion, AppDynamics’ approach to MLOps is revolutionizing the way companies monitor and observe their applications. By leveraging machine learning models, AppDynamics is able to provide real-time, actionable insights into the performance of your application and machine learning models. Whether you’re looking to improve fraud detection or implement predictive maintenance, AppDynamics’ MLOps platform has you covered. So what are you waiting for? Start leveraging the power of MLOps with AppDynamics today!