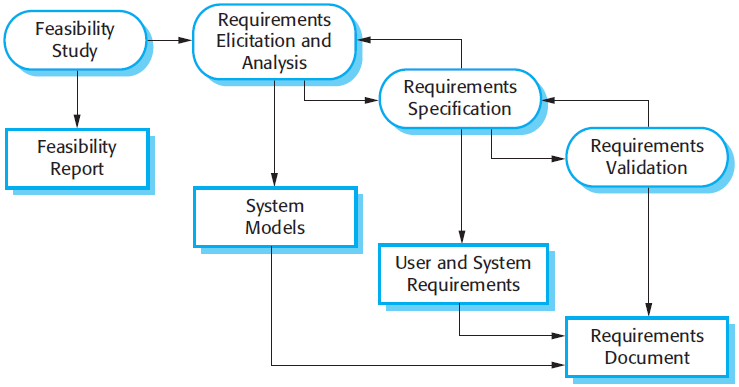
Have you ever wondered what sets MLOps and ITOps apart? While they may sound similar, they serve different purposes in the world of technology. In this article, we will dive into the key differences between MLOps and ITOps.
MLOps: What it is and How it Works
MLOps, short for Machine Learning Operations, is the practice of applying DevOps principles to machine learning. It involves managing the entire machine learning lifecycle, from data preparation to model deployment.
The goal of MLOps is to streamline the development and deployment of machine learning models, making it easier for organizations to incorporate machine learning into their operations. This involves automating processes and creating a standardized approach to machine learning development.
MLOps also involves collaboration between different teams, including data scientists, developers, and operations teams. By working together, they can ensure that the machine learning model is developed and deployed efficiently and effectively.
ITOps: What it is and How it Works
ITOps, short for IT Operations, is the practice of managing the infrastructure and systems that support an organization’s IT services. This includes managing servers, networks, and other hardware and software components.
The goal of ITOps is to ensure that the IT infrastructure is reliable, secure, and efficient. This involves monitoring and maintaining the infrastructure, as well as troubleshooting any issues that arise.
ITOps also involves collaboration between different teams, including system administrators, network engineers, and security professionals. By working together, they can ensure that the IT infrastructure is running smoothly and securely.
Key Differences Between MLOps and ITOps
While MLOps and ITOps both involve managing technology, there are several key differences between them.
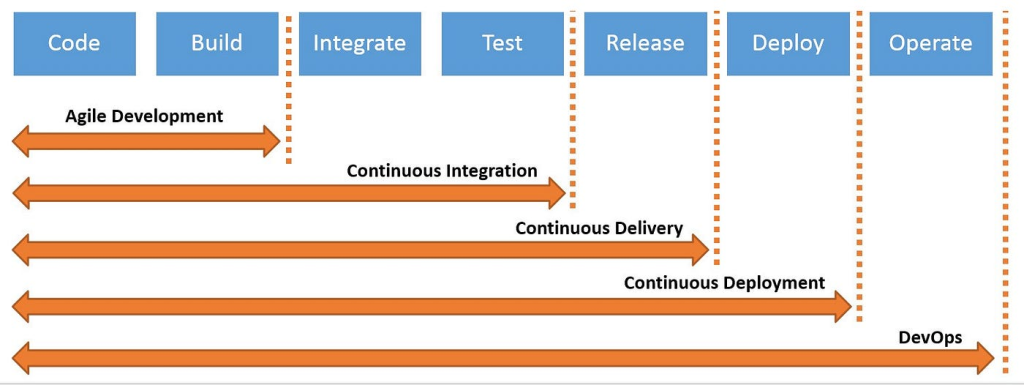
Focus
MLOps focuses on the development and deployment of machine learning models, while ITOps focuses on managing the IT infrastructure that supports an organization’s IT services.
Teams Involved
MLOps involves collaboration between data scientists, developers, and operations teams, while ITOps involves collaboration between system administrators, network engineers, and security professionals.
Processes Involved
MLOps involves automating processes and creating a standardized approach to machine learning development, while ITOps involves monitoring and maintaining the IT infrastructure.
Goals
The goal of MLOps is to streamline the development and deployment of machine learning models, while the goal of ITOps is to ensure that the IT infrastructure is reliable, secure, and efficient.
Conclusion
In conclusion, while MLOps and ITOps may sound similar, they serve different purposes in the world of technology. MLOps focuses on the development and deployment of machine learning models, while ITOps focuses on managing the IT infrastructure that supports an organization’s IT services. By understanding the key differences between MLOps and ITOps, organizations can better leverage these practices to improve their technology operations.