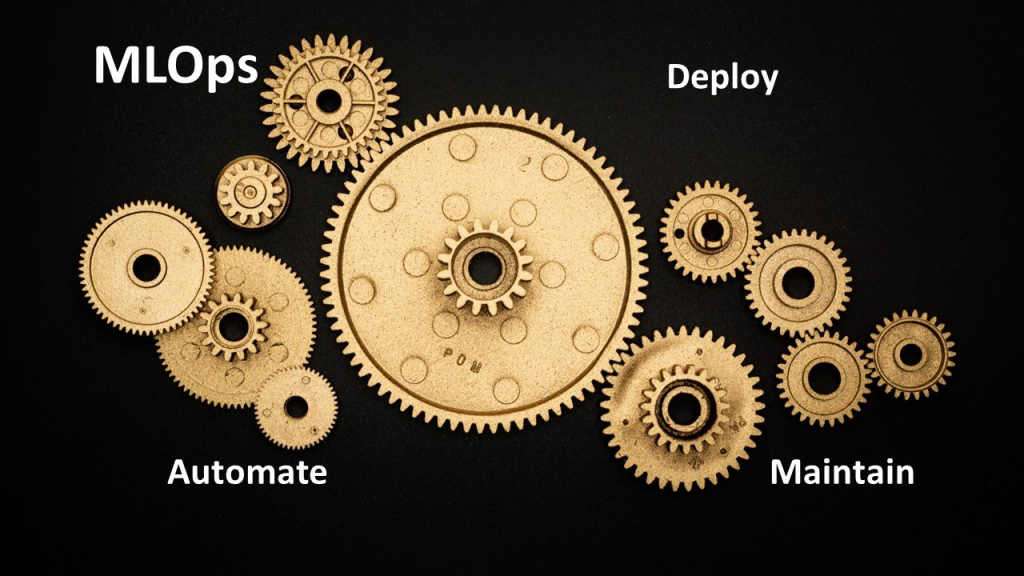
Are you tired of hearing buzzwords like “MLOps” thrown around without really understanding what they mean? Fear not, dear reader, for we are here to demystify this concept and explore its purpose.
Introduction: The Rise of MLOps
Machine learning has rapidly evolved in recent years, with companies across various industries leveraging it to gain a competitive edge. However, as models become more complex and data sets grow, managing the entire machine learning lifecycle can become a daunting task.
This is where MLOps comes in. MLOps, or “Machine Learning Operations,” is a set of best practices and tools that aim to streamline the deployment, maintenance, and scaling of machine learning models.
But what is the ultimate goal of MLOps? Let’s dive in.
Goal 1: Faster Time to Market
One of the primary objectives of MLOps is to speed up the time it takes to get a machine learning model into production. Traditionally, the process of developing and deploying a model can take months, involving multiple teams and manual processes.
MLOps aims to automate many of these steps, such as data preprocessing, model training, and deployment, to reduce the time it takes to get a model into production. This faster time to market can give companies a significant advantage in a constantly evolving market.
Goal 2: Improved Model Performance
Another crucial goal of MLOps is to improve the performance of machine learning models. In many cases, models that perform well in a development environment may not perform as well in a production environment.
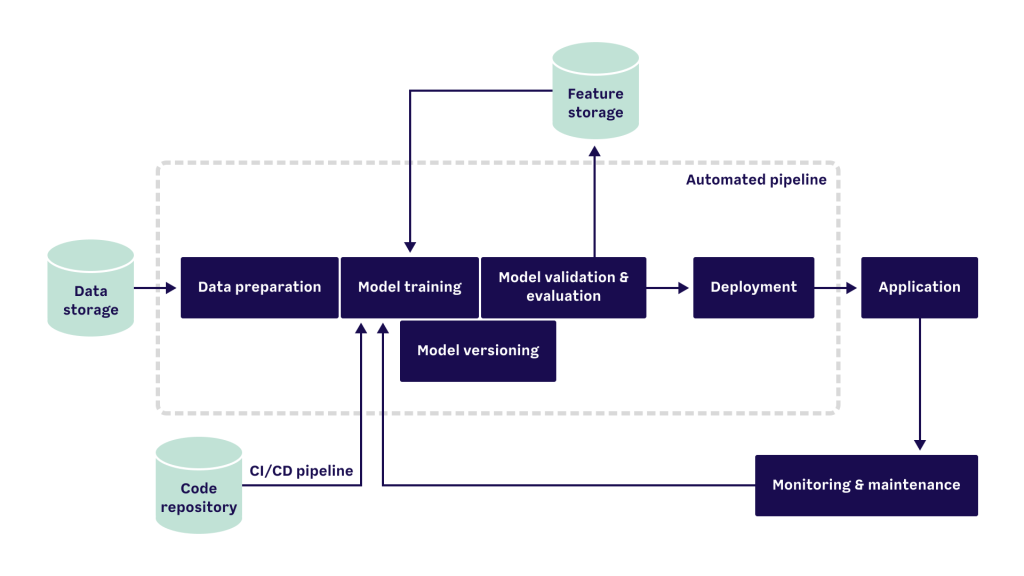
MLOps helps address this issue by providing tools for monitoring and optimizing models in real-time. This can include setting up alerts for model performance degradation, automatically retraining models with new data, and deploying models to multiple environments to ensure consistent performance.
Goal 3: Increased Collaboration
Finally, MLOps aims to promote collaboration between different teams involved in the machine learning lifecycle. This includes data scientists, developers, and operations teams.
By breaking down silos and promoting transparency, MLOps can help ensure that everyone is working towards the same goal. This can lead to better communication, faster feedback loops, and ultimately, better models.
Conclusion: The Importance of MLOps
In conclusion, the goal of MLOps is to improve the efficiency, performance, and collaboration of the entire machine learning lifecycle. By automating many of the manual processes involved in model development and deployment, companies can speed up their time to market, improve model performance, and foster better collaboration between teams.
So the next time you hear the term “MLOps,” remember that it’s not just another buzzword. It’s a critical component of a successful machine learning strategy.