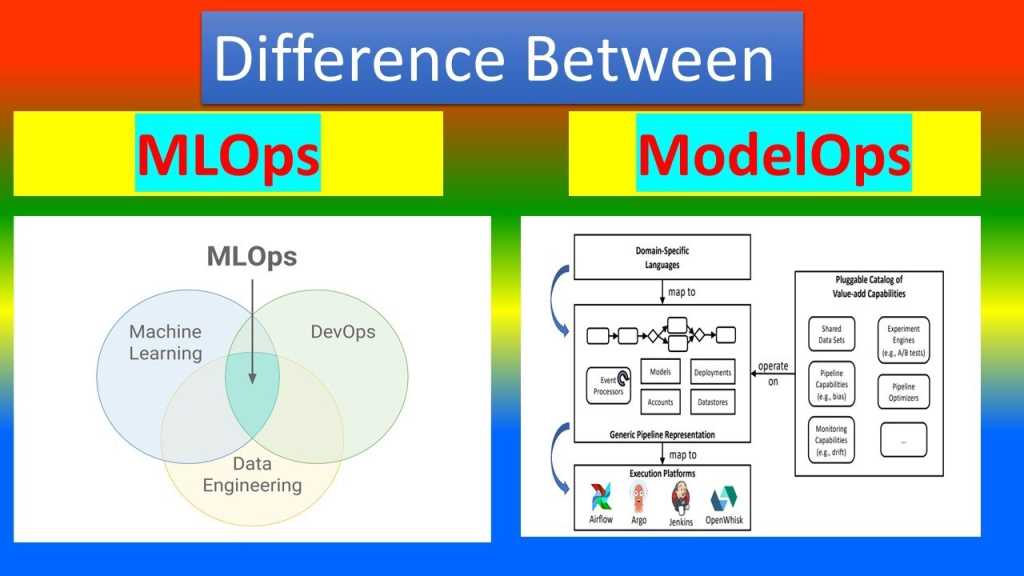
As the world of technology continues to evolve, new concepts and buzzwords emerge, making it challenging to keep up with the latest trends. One of the latest buzzwords that have been making rounds in the technology world is MLOps and mlops. But what do these terms mean, and what sets them apart? In this blog post, we will explore the significant differences between MLOps and mlops.
Defining MLOps and mlops
MLOps, which stands for Machine Learning Operations, refers to the process of managing and deploying machine learning models. It involves the use of automated tools and workflows to streamline the development, testing, and deployment of machine learning models. On the other hand, mlops, which stands for machine learning operations, is a term used to describe the operationalization of machine learning models.
The Major Differences Between MLOps vs mlops
While MLOps and mlops may sound similar, they differ in several ways, including:
1. Scope
The scope of MLOps is more extensive than that of mlops. MLOps encompasses the entire machine learning development lifecycle, from data preparation to model deployment and monitoring. It also includes the use of various tools and processes to automate the development and deployment of machine learning models. On the other hand, mlops focuses mainly on the operationalization of machine learning models, which involves deploying and managing them in a production environment.
2. Complexity
MLOps is more complex than mlops. This is because it involves a more extensive set of processes, tools, and workflows. It also requires a more significant investment in terms of time, resources, and expertise. MLOps requires a team of data scientists, software engineers, and DevOps experts to work together to ensure that the machine learning model is developed, tested, and deployed efficiently. In contrast, mlops involves deploying pre-trained machine learning models and managing them in a production environment, which is less complex than MLOps.
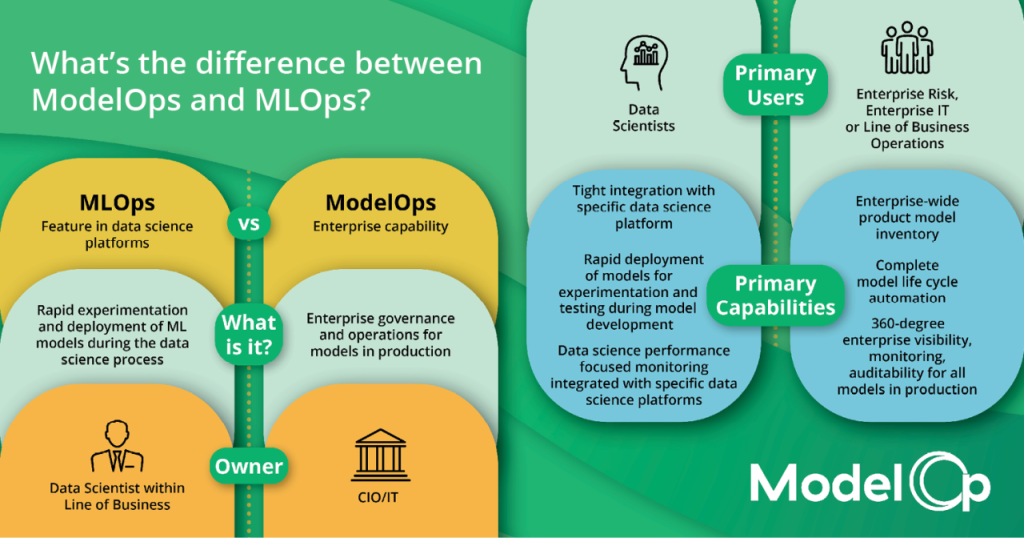
3. Technology Stack
MLOps and mlops also differ in terms of the technology stack used. MLOps uses a variety of tools and technologies, including data preparation tools, machine learning frameworks, and deployment tools. These tools are used to automate the development and deployment of machine learning models. In contrast, mlops uses a narrower set of tools and technologies, mainly focusing on deploying and managing pre-trained machine learning models in a production environment.
Conclusion
In conclusion, while MLOps and mlops may sound similar, they differ significantly in terms of scope, complexity, and technology stack. MLOps is a more comprehensive approach that encompasses the entire machine learning development lifecycle. It involves the use of various tools and workflows to automate the development, testing, and deployment of machine learning models. On the other hand, mlops focuses mainly on the operationalization of machine learning models, which involves deploying and managing pre-trained models in a production environment.